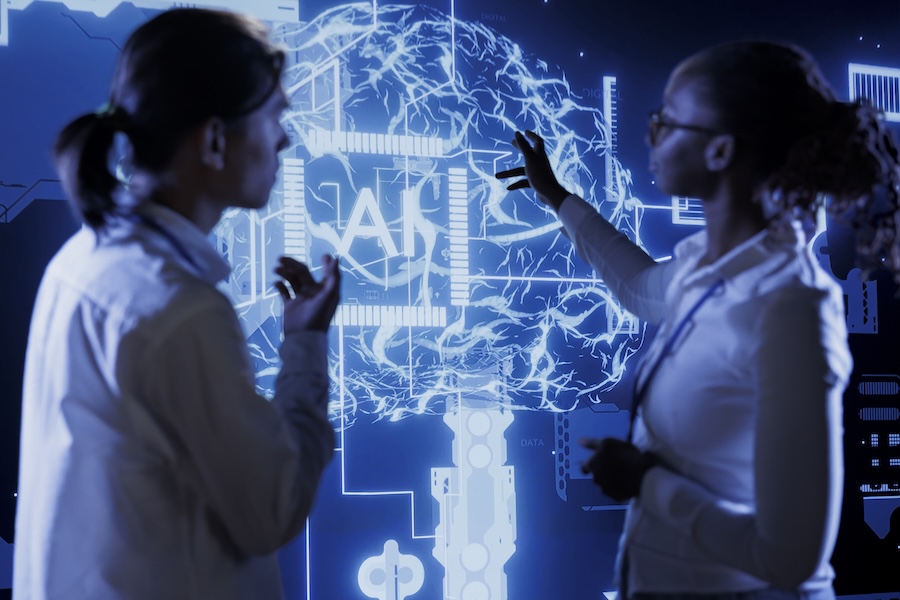
Designing, Developing and Deploying AI-Assisted Patient Communication Solutions
Design and Development Phase
The design and development of AI-assisted patient communication and support solutions should begin with a human-centred approach that prioritises understanding user needs and contextual factors. The first stage is to design and develop AI solutions for the right problems using a human-centred AI and experimentation approach, engaging appropriate stakeholders, especially the healthcare users themselves^5. This approach requires building a multidisciplinary team including computer and social scientists, operational and research leadership, clinical stakeholders (physicians, caregivers, and patients), and subject experts who can provide diverse perspectives and expertise^5. Such collaboration ensures that the AI solution addresses genuine needs within healthcare settings rather than simply applying technology for its own sake.
Through user-designed research, developers should first understand the key problems by conducting qualitative studies to determine what the problem is, why it is a problem, to whom it matters, why it has not been addressed before, and why it is not getting attention^5. After defining key problems, the next step is to identify which problems are appropriate for AI to solve and whether there is availability of applicable datasets to build and later evaluate the AI solution^5. By contextualising algorithms in existing workflows, AI systems would operate within established norms and practices, ensuring adoption and providing appropriate solutions for end users. The development process should embrace experimentation with tight feedback loops from stakeholders to facilitate rapid experiential learning and incremental improvements^5. This iterative approach allows for continuous refinement of the AI solution based on real-world feedback.
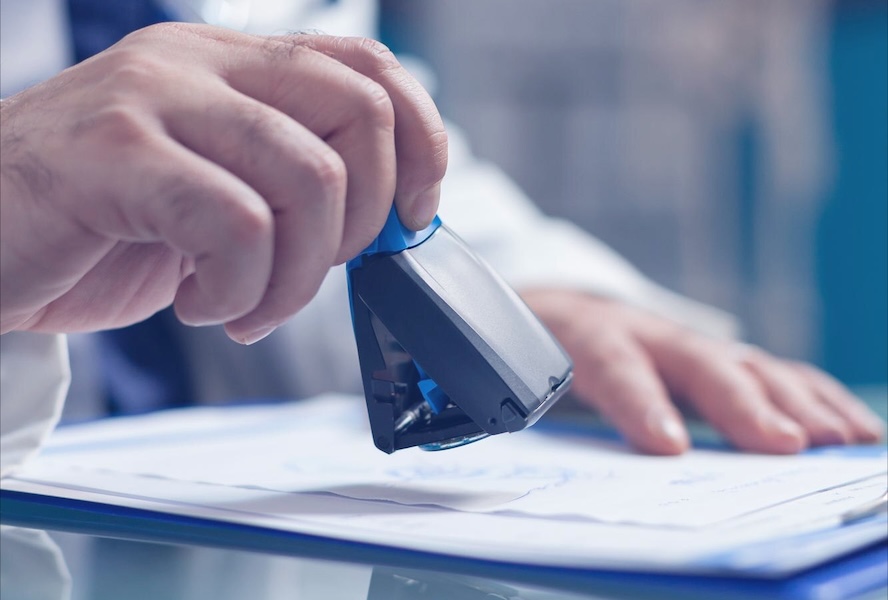
Classification > Purpose >
Validation > Documentation
Regulatory Considerations
When developing AI-assisted patient communication and support solutions, understanding and addressing regulatory requirements from the outset is crucial for successful deployment. Initially, developers must determine whether their solution qualifies as a medical device under relevant regulatory frameworks^1^6. This determination depends primarily on the intended purpose of the AI system, particularly whether it is used for diagnosis, prevention, monitoring, prognosis, treatment, or alleviation of disease^1. If the AI solution falls within the definition of a medical device, developers must proceed with appropriate regulatory pathways, which vary by jurisdiction but typically involve registration with regulatory bodies such as the TGA in Australia, MHRA in the UK, or FDA in the US^1^4.
Once classified as a medical device, risk classification becomes a critical consideration that determines the level of regulatory scrutiny^3. Developers must formulate a precise intended purpose for their AI solution, as required by regulators like the MDR/IVDR in the EU^6. This intended purpose must be clearly articulated and will serve as the foundation for validation activities. Manufacturers must validate their devices against this intended purpose and stakeholder requirements, as well as verify them against specifications^6. Additionally, developers need to prepare comprehensive documentation describing the methods used to provide evidence of safety and effectiveness, which is essential for regulatory submissions^6. These regulatory considerations should be integrated into the development process from the beginning rather than treated as an afterthought, as this proactive approach can prevent costly redesigns and delays.
Testing and Validation
Comprehensive testing and validation are essential components in the development lifecycle of AI-assisted patient communication solutions to ensure they meet both regulatory requirements and user needs. Testing should encompass technical performance, clinical validity, and usability aspects, with validation activities designed to demonstrate that the AI solution achieves its intended purpose safely and effectively^6. Quality management system principles should be applied throughout the development process, embedding risk management, documentation, configuration management, and measurement into product planning^10. These quality processes help ensure systematic and consistent approaches to development and testing, which are particularly important given the complex and often opaque nature of AI systems.
Developers should ensure their AI solutions demonstrate safety, security, and robustness under various conditions, including edge cases and potential failure modes^2. Appropriate transparency and explainability mechanisms should be incorporated to allow users to understand how the AI arrives at recommendations or decisions, which is particularly important in healthcare settings where clinicians remain ultimately responsible for patient care^2. User-centred design approaches should continue through the testing phase, with usability evaluations involving actual end-users such as healthcare professionals and patients^13. A study on designing interfaces to communicate AI-based prognosis found that clinicians requested enhancements that emphasised interpretability over explainability, highlighting the importance of translating complex AI outputs into clinically meaningful information^13. These findings underscore the need for testing that focuses not just on technical accuracy but also on how well the AI solution integrates into clinical workflows and supports meaningful human-AI collaboration.
Deployment and Post-Market Surveillance
Successful deployment of AI-assisted patient communication solutions extends beyond initial market introduction to encompass ongoing monitoring, maintenance, and improvement throughout the product lifecycle. Post-market surveillance is a regulatory requirement across jurisdictions and involves systematic collection and analysis of real-world performance data to ensure continued safety and effectiveness^4. Developers should implement robust monitoring systems to track the performance of their AI solutions in diverse clinical settings and patient populations, enabling early detection of any unexpected issues or performance degradation^4. This ongoing vigilance is particularly important for AI systems that may encounter novel data patterns or operational environments not represented in training or validation datasets.
Risk management should continue throughout the deployment phase, with processes in place to identify, assess, and mitigate emerging risks^10. Plans for maintenance and updates should be established, including protocols for algorithm retraining or refinement based on new data^10. The FDA has issued guidance on predetermined change control plans for AI-enabled devices, which provides recommendations on how to proactively plan for device updates once the product is on the market^4. User education and training are equally important aspects of deployment, ensuring that healthcare professionals understand both the capabilities and limitations of the AI solution^11. The European Patients’ Forum has advocated for AI solutions in healthcare that uphold principles of patient safety, transparency, privacy, human autonomy, co-design, accountability, and education^11. These principles emphasise that successful deployment depends not only on technical performance but also on fostering trust and understanding among users, ultimately leading to better integration of AI technologies into healthcare practice and improved patient outcomes.
Conclusion
The development of AI in healthcare represents a significant opportunity to improve patient care, enhance clinical decision-making, and increase healthcare system efficiency. However, realising these benefits requires thoughtful consideration of both technical and human factors throughout the development process.
By engaging multidisciplinary teams, conducting iterative experimentation with user feedback, ensuring regulatory compliance, and maintaining vigilance through post-market monitoring, developers can create AI solutions that genuinely enhance patient communication and support while navigating the complex regulatory landscape. As regulatory frameworks continue to mature and clinical experience with AI expands, we can expect greater harmonisation of approaches and clearer pathways for bringing beneficial AI technologies to patients while maintaining the high standards of safety and effectiveness that healthcare demands.
Don’t Miss Part 1 – AI-Driven Patient Communications – The Regulations
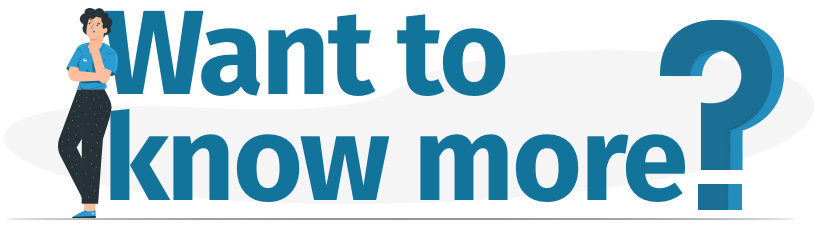
Organisations: We’re offering a free 30-minute, no-obligation call for organisations interested in supercharging their patient communications and keeping their multidisciplinary care teams in the loop with patient progress. Check our calendar here for a free spot.
Doctors: Health professionals can use the PEP Health platform for free. Talk with us now to find out how.
References
- MinterEllison. (2024). Innovation meets regulation: Medical devices and artificial intelligence. Retrieved from https://www.minterellison.com/articles/innovation-meets-regulation-medical-devices-and-artificial-intelligence^1
- Medicines and Healthcare products Regulatory Agency. (2024). MHRA’s AI regulatory strategy ensures patient safety and industry innovation into 2030. Retrieved from https://www.gov.uk/government/news/mhras-ai-regulatory-strategy-ensures-patient-safety-and-industry-innovation-into-2030^2
- VDE. (2024). Approval of AI-based medical devices in Europe. Retrieved from https://www.vde.com/topics-en/health/consulting/approval-of-ai-based-medical-devices-in-europe^3
- U.S. Food and Drug Administration. (2025). FDA Issues Comprehensive Draft Guidance for Developers of Artificial Intelligence-Enabled Medical Devices. Retrieved from https://www.fda.gov/news-events/press-announcements/fda-issues-comprehensive-draft-guidance-developers-artificial-intelligence-enabled-medical-devices^4
- Staes et al. (2021). Artificial intelligence in healthcare: transforming the practice of medicine. Retrieved from https://pmc.ncbi.nlm.nih.gov/articles/PMC8285156/^5
- Johner Institute. (2025). Regulatory requirements for medical devices with machine learning. Retrieved from https://blog.johner-institute.com/regulatory-affairs/regulatory-requirements-for-medical-devices-with-machine-learning/^6
- Research Australia. (2022). Artificial Intelligence and Automated Decision making. Retrieved from https://researchaustralia.org/wp-content/uploads/2022/05/Research-Aust-Sub-AI-and-ADM-Regulation-FINAL.pdf^7
- Inside EU Life Sciences. (2024). MHRA Outlines New Strategic Approach to Artificial Intelligence. Retrieved from https://www.insideeulifesciences.com/2024/05/01/mhra-outlines-new-strategic-approach-to-artificial-intelligence/^8
- Health Action International. (2023). The EU Medical Devices Regulation and the EU AI Act: A Short Comparison. Retrieved from https://haiweb.org/wp-content/uploads/2023/03/MDR-AIAct_OnePager_FINAL.pdf^9
- Ronaldson et al. (2020). Regulatory Frameworks for Development and Evaluation of Artificial Intelligence-Based Diagnostic Imaging Algorithms: Summary and Recommendations. Retrieved from https://pmc.ncbi.nlm.nih.gov/articles/PMC7574690/^10
- European Patients’ Forum. (2025). AI in Healthcare: Advancing Patient-Centric Care through Co-design and Responsible Implementation. Retrieved from https://www.eu-patient.eu/news/latest-epf-news/2023/artificial-intai-in-healthcare-advancing-patient-centric-care-through-co-design-and-responsible-implementation/^11
- Bayes et al. (2022). Using Existing Regulatory Frameworks to Apply Effective Design Controls to AI/ML-Enabled Medical Devices. Retrieved from https://pmc.ncbi.nlm.nih.gov/articles/PMC10512990/^12
- Staes et al. (2023). Design of an interface to communicate artificial intelligence-based prognosis for patients with advanced solid tumors: a user-centered approach. Journal of the American Medical Informatics Association, 31(1), 174-187. Retrieved from https://academic.oup.com/jamia/article/31/1/174/7320060^13
Recent Comments